Bibcode
Mong, Y. -L.; Ackley, K.; Galloway, D. K.; Killestein, T.; Lyman, J.; Steeghs, D.; Dhillon, V.; O'Brien, P. T.; Ramsay, G.; Poshyachinda, S.; Kotak, R.; Nuttall, L.; Pallé, E.; Pollacco, D.; Thrane, E.; Dyer, M. J.; Ulaczyk, K.; Cutter, R.; McCormac, J.; Chote, P.; Levan, A. J.; Marsh, T.; Stanway, E.; Gompertz, B.; Wiersema, K.; Chrimes, A.; Obradovic, A.; Mullaney, J.; Daw, E.; Littlefair, S.; Maund, J.; Makrygianni, L.; Burhanudin, U.; Starling, R. L. C.; Eyles-Ferris, R. A. J.; Tooke, S.; Duffy, C.; Aukkaravittayapun, S.; Sawangwit, U.; Awiphan, S.; Mkrtichian, D.; Irawati, P.; Mattila, S.; Heikkilä, T.; Breton, R.; Kennedy, M.; Mata Sánchez, D.; Rol, E.
Referencia bibliográfica
Monthly Notices of the Royal Astronomical Society
Fecha de publicación:
10
2020
Número de citas
12
Número de citas referidas
9
Descripción
The amount of observational data produced by time-domain astronomy is exponentially increasing. Human inspection alone is not an effective way to identify genuine transients from the data. An automatic real-bogus classifier is needed and machine learning techniques are commonly used to achieve this goal. Building a training set with a sufficiently large number of verified transients is challenging, due to the requirement of human verification. We present an approach for creating a training set by using all detections in the science images to be the sample of real detections and all detections in the difference images, which are generated by the process of difference imaging to detect transients, to be the samples of bogus detections. This strategy effectively minimizes the labour involved in the data labelling for supervised machine learning methods. We demonstrate the utility of the training set by using it to train several classifiers utilizing as the feature representation the normalized pixel values in 21 × 21 pixel stamps centred at the detection position, observed with the Gravitational-wave Optical Transient Observer (GOTO) prototype. The real-bogus classifier trained with this strategy can provide up to $95{{\ \rm per\ cent}}$ prediction accuracy on the real detections at a false alarm rate of $1{{\ \rm per\ cent}}$ .
Proyectos relacionados
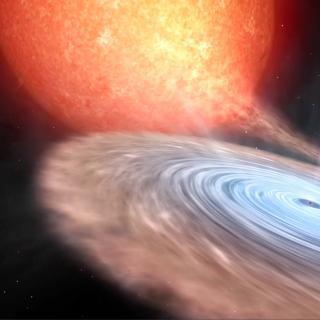
Agujeros negros, estrellas de neutrones, enanas blancas y su entorno local
Los agujeros negros y estrellas de neutrones en binarias de rayos-X son laboratorios únicos para explorar la física de estos objetos compactos. No solo permiten confirmar la existencia de agujeros negros de origen estelar a través de mediciones dinámicas de sus masas, sino que también permiten investigar el comportamiento de la materia y la
Montserrat
Armas Padilla
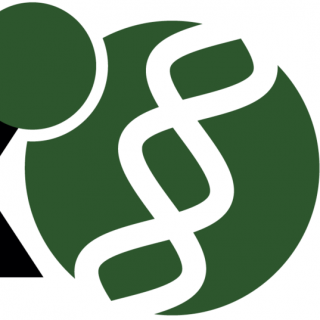
Exoplanetas y Astrobiología
La búsqueda de vida en el Universo se ha visto impulsada por los recientes descubrimientos de planetas alrededor de otras estrellas (los llamados exoplanetas), convirtiéndose en uno de los campos más activos dentro de la Astrofísica moderna. En los últimos años los descubrimientos cada vez más numerosos de nuevos exoplanetas y los últimos avances
Enric
Pallé Bago