Bibcode
Asensio Ramos, A.
Bibliographical reference
Astronomy and Astrophysics
Advertised on:
8
2024
Journal
Citations
0
Refereed citations
0
Description
Context. The study of astronomical phenomena through ground-based observations is always challenged by the distorting effects of Earth's atmosphere. Traditional methods of post facto image correction, essential for correcting these distortions, often rely on simplifying assumptions that limit their effectiveness, particularly in the presence of spatially variant atmospheric turbulence. Such cases are often solved by partitioning the field of view into small patches, deconvolving each patch independently, and merging all patches together. This approach is often inefficient and can produce artifacts.
Aims: Recent advancements in computational techniques and the advent of deep learning offer new pathways to address these limitations. This paper introduces a novel framework leveraging a deep neural network to emulate spatially variant convolutions, offering a breakthrough in the efficiency and accuracy of astronomical image deconvolution.
Methods: By training on a dataset of images convolved with spatially invariant point spread functions and validating its generalizability to spatially variant conditions, this approach presents a significant advancement over traditional methods. The convolution emulator is used as a forward model in a multiobject multiframe blind deconvolution algorithm for solar images.
Results: The emulator enables the deconvolution of solar observations across large fields of view without resorting to patch-wise mosaicking, thus avoiding the artifacts associated with such techniques. This method represents a significant computational advantage, reducing processing times by orders of magnitude.
Aims: Recent advancements in computational techniques and the advent of deep learning offer new pathways to address these limitations. This paper introduces a novel framework leveraging a deep neural network to emulate spatially variant convolutions, offering a breakthrough in the efficiency and accuracy of astronomical image deconvolution.
Methods: By training on a dataset of images convolved with spatially invariant point spread functions and validating its generalizability to spatially variant conditions, this approach presents a significant advancement over traditional methods. The convolution emulator is used as a forward model in a multiobject multiframe blind deconvolution algorithm for solar images.
Results: The emulator enables the deconvolution of solar observations across large fields of view without resorting to patch-wise mosaicking, thus avoiding the artifacts associated with such techniques. This method represents a significant computational advantage, reducing processing times by orders of magnitude.
Related projects
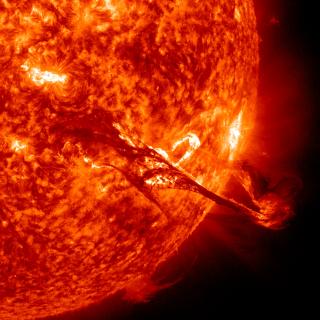
Solar and Stellar Magnetism
Magnetic fields are at the base of star formation and stellar structure and evolution. When stars are born, magnetic fields brake the rotation during the collapse of the mollecular cloud. In the end of the life of a star, magnetic fields can play a key role in the form of the strong winds that lead to the last stages of stellar evolution. During
Tobías
Felipe García