The identification of active regions in the far-side of the Sun by analyzing the oscillations on the Earth-side is one of the most impressive results from local helioseismology. The interest in the detection of active regions in the farside goes beyond the simple curiosity of measuring them before they rotate into the visible hemisphere. Due to the importance of the far-side magnetism for solar studies and space weather predictions, one would expect that in the future there will be telescopes permanently observing the whole Sun. While this future arrives, the only method capable of constantly monitoring the solar far-side is helioseismology.
Far-side helioseismology is challenged by the low signal-to-noise. Only large and strong active regions can be reliably detected in farside phase-shift maps. In this project, we aim to develop new methods to detect the presence of active regions in the non-visible solar hemisphere. Our approach will be based on the use of machine learning tools.
Due to the growing interest in the young field of research of space weather and its fundamental scientific, social, and economic impact, far-side helioseismology will have a key role in the forthcoming decades. This project will tackle the main limitations of far-side helioseismology, and will significantly enhance its practical applications.
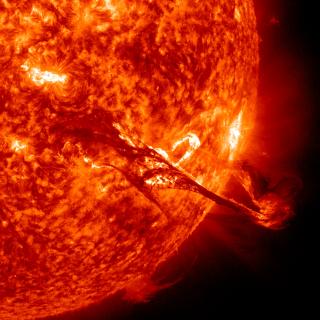