Bibcode
Killestein, T. L.; Kelsey, L.; Wickens, E.; Nuttall, L.; Lyman, J.; Krawczyk, C.; Ackley, K.; Dyer, M. J.; Jiménez-Ibarra, F.; Ulaczyk, K.; O'Neill, D.; Kumar, A.; Steeghs, D.; Galloway, D. K.; Dhillon, V. S.; O'Brien, P.; Ramsay, G.; Noysena, K.; Kotak, R.; Breton, R. P.; Pallé, E.; Pollacco, D.; Awiphan, S.; Belkin, S.; Chote, P.; Clark, P.; Coppejans, D.; Duffy, C.; Eyles-Ferris, R.; Godson, B.; Gompertz, B.; Graur, O.; Irawati, P.; Jarvis, D.; Julakanti, Y.; Kennedy, M. R.; Kuncarayakti, H.; Levan, A.; Littlefair, S.; Magee, M.; Mandhai, S.; Mata Sánchez, D.; Mattila, S.; McCormac, J.; Mullaney, J.; Munday, J.; Patel, M.; Pursiainen, M.; Rana, J.; Sawangwit, U.; Stanway, E.; Starling, R.; Warwick, B.; Wiersema, K.
Bibliographical reference
Monthly Notices of the Royal Astronomical Society
Advertised on:
9
2024
Citations
0
Refereed citations
0
Description
Time-domain astrophysics continues to grow rapidly, with the inception of new surveys drastically increasing data volumes. Democratized, distributed approaches to training sets for machine learning classifiers are crucial to make the most of this torrent of discovery - with citizen science approaches proving effective at meeting these requirements. In this paper, we describe the creation of and the initial results from the Kilonova Seekers citizen science project, built to find transient phenomena from the GOTO telescopes in near real-time. Kilonova Seekers launched in 2023 July and received over 600 000 classifications from approximately 2000 volunteers over the course of the LIGO-Virgo-KAGRA O4a observing run. During this time, the project has yielded 20 discoveries, generated a 'gold-standard' training set of 17 682 detections for augmenting deep-learned classifiers, and measured the performance and biases of Zooniverse volunteers on real-bogus classification. This project will continue throughout the lifetime of GOTO, pushing candidates at ever-greater cadence, and directly facilitate the next-generation classification algorithms currently in development.
Related projects
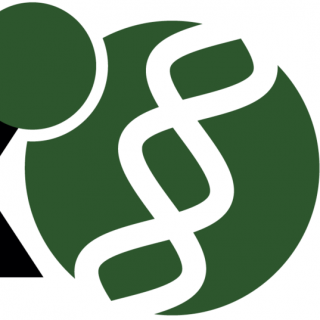
Exoplanets and Astrobiology
The search for life in the universe has been driven by recent discoveries of planets around other stars (known as exoplanets), becoming one of the most active fields in modern astrophysics. The growing number of new exoplanets discovered in recent years and the recent advance on the study of their atmospheres are not only providing new valuable
Enric
Pallé Bago
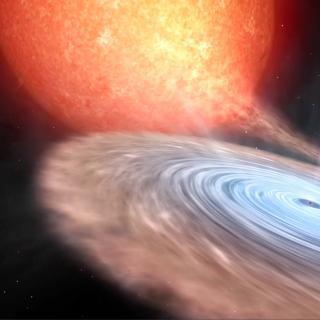
Black holes, neutron stars, white dwarfs and their local environment
Accreting black-holes and neutron stars in X-ray binaries provide an ideal laboratory for exploring the physics of compact objects, yielding not only confirmation of the existence of stellar mass black holes via dynamical mass measurements, but also the best opportunity for probing high-gravity environments and the physics of accretion; the most
Montserrat
Armas Padilla